How to Hack AI?
Machine Learning Vulnerabilities That Nobody Talks About
As machine learning (ML) systems become a staple of everyday life, the security threats they entail will spill over into all kinds of applications we use, according to a new report.
Unlike traditional software, where flaws in design and source code account for most security issues, in AI systems, vulnerabilities can exist in images, audio files, text, and other data used to train and run machine learning models.
The problem of finding software vulnerabilities seems well-suited for ML systems. Going through code line by line is just the sort of tedious problem that computers excel at, if we can only teach them what a vulnerability looks like. There are challenges with that, of course, but there is already a healthy amount of academic literature on the topic and research is continuing. There’s every reason to expect ML systems to get better at this as time goes on, and some reason to expect them to eventually become very good at it.
With artificial intelligence used for consequential applications, concerns grow about security. Long ago were days when Deep Blue beat Gary Kasparov in a chess match, and it was considered a significant breakthrough. If AI makes a mistake in a recommendation system in the retail industry, it is tolerable. However, if a self-driving car makes a mistake, it can even lead to people getting injured or losing lives. So how to hack AI? In this article, we will discuss the vulnerabilities of machine learning.
The gravity of these AI applications calls for an extensive talk on machine learning security. A machine learning algorithm can propagate itself in many ways. People with malicious intentions can recognize specific flaws in an AI system. If they do that before data scientists who created it, the results can be catastrophic. Data science and cybersecurity tasks are to develop solutions to counter these attacks.
AI Security Problems
Artificial intelligence security problems often manifest themselves as exploitations of machine learning techniques. The algorithms are tricked into making a wrong assessment of the whole situation. When the input is wrong, the output contains wrong, or even fatal decisions. AI/ML systems hold the same opportunities for exploitation and misconfigurations as any other technology, but it also has its own unique risks. As more enterprises focus on major AI-powered digital transformations, those risks only grow in vulnerability.
There are 2 critical assets in AI. The first is data, big data. This is how AI can learn/train and infer its predictions and insights. The second asset is the data model itself. The model is the result of training the algorithm with big data and this is a competitive edge of every company. Data driven companies should design a solution to secure both big data and data models to protect their AI projects.
Since artificial intelligence and machine learning systems require higher volumes of complex data, there are many ways in which AI/ML systems can be exploited. While there are mechanisms in place for detecting and thwarting attacks that organizations can leverage, the question remains, how to leverage existing principles for confidential computing in order to develop secure AI applications.
There are already four areas where AI attacks would deal the most damage:
Military/police forces
AI-powered robots replacing human tasks
Civil society
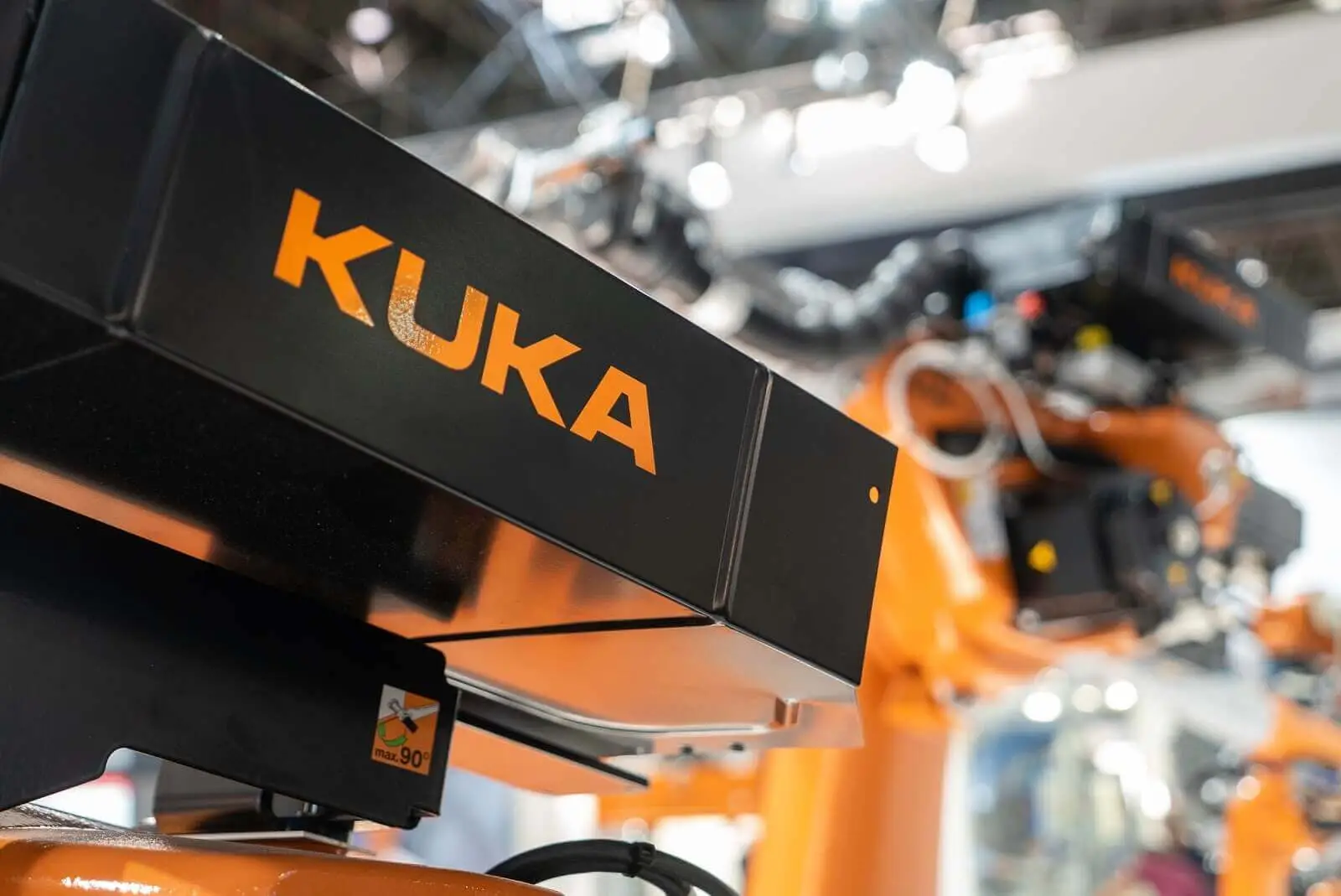
AI handles 75% network security solutions in international enterprises. Gartner predicts that the expenses related to cybersecurity and risk management will be as high as $175.5 billion by 2023.
With AI in charge of security solutions in IT, fooling these algorithms like circumventing security. It’s the reason why cybersecurity experts and data scientists need to work together to prevent that from happening. It’s like surprising YouTube’s recommendation systems by listening to a new genre of music!
Tricking Tesla Model S
For example, conveniently placed stickers managed to trick a Tesla Model S into recognizing a stop sign as an “Added Lane” sign. In any traffic, that would most likely crash it. Also, stickers managed to trick its algorithm into seeing 85 instead of 35 on a speed limit sign. Check out the modified sign on the figure below!
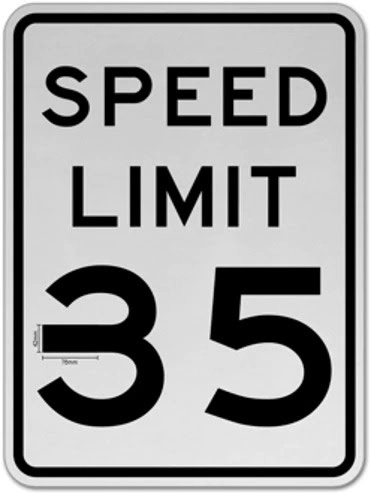
Credits: Model Hacking ADAS to Pave Safer Roads for Autonomous Vehicles
The human brain would effortlessly deduce that the speed limit is 35. But, machine learning algorithms still struggle with samples that deviate from the majority. This example might be synthetic, but it shows how damaged traffic signs represent potential AI's weak spot.
Video Surveillance Fails
In most cases, machine learning algorithms can be fooled by manipulating the underlying pixels of an image. These changes are invisible to humans, but they lead the algorithm astray. Take a look below to see how the methods can make AI see a helicopter on a photo with four automatic weapons:
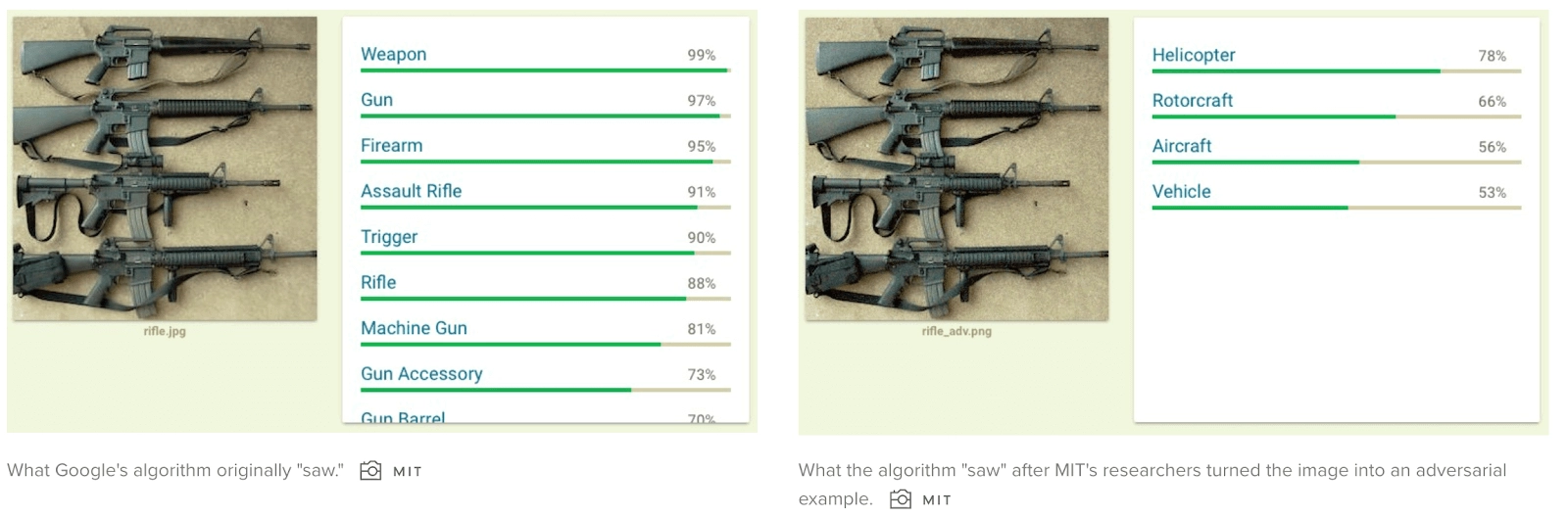
Credits: Is Fooling An AI Algorithm Really That Easy?
In some cases, you can fool these algorithms without using technology or image editing. Below you can see that the person on the left is recognized, but the person on the right, wearing a painting on his torso, isn’t. Machine learning techniques didn’t expect this, and it has led to the wrong decision. Deep learning/machine learning models rely on analyzing user behavior. Here is what happens when you don’t follow what the algorithm perceives as normal user behavior. Remember that AI-powered image recognition is also used in medicine. Exploiting its vulnerabilities might lead to a wrong diagnosis.
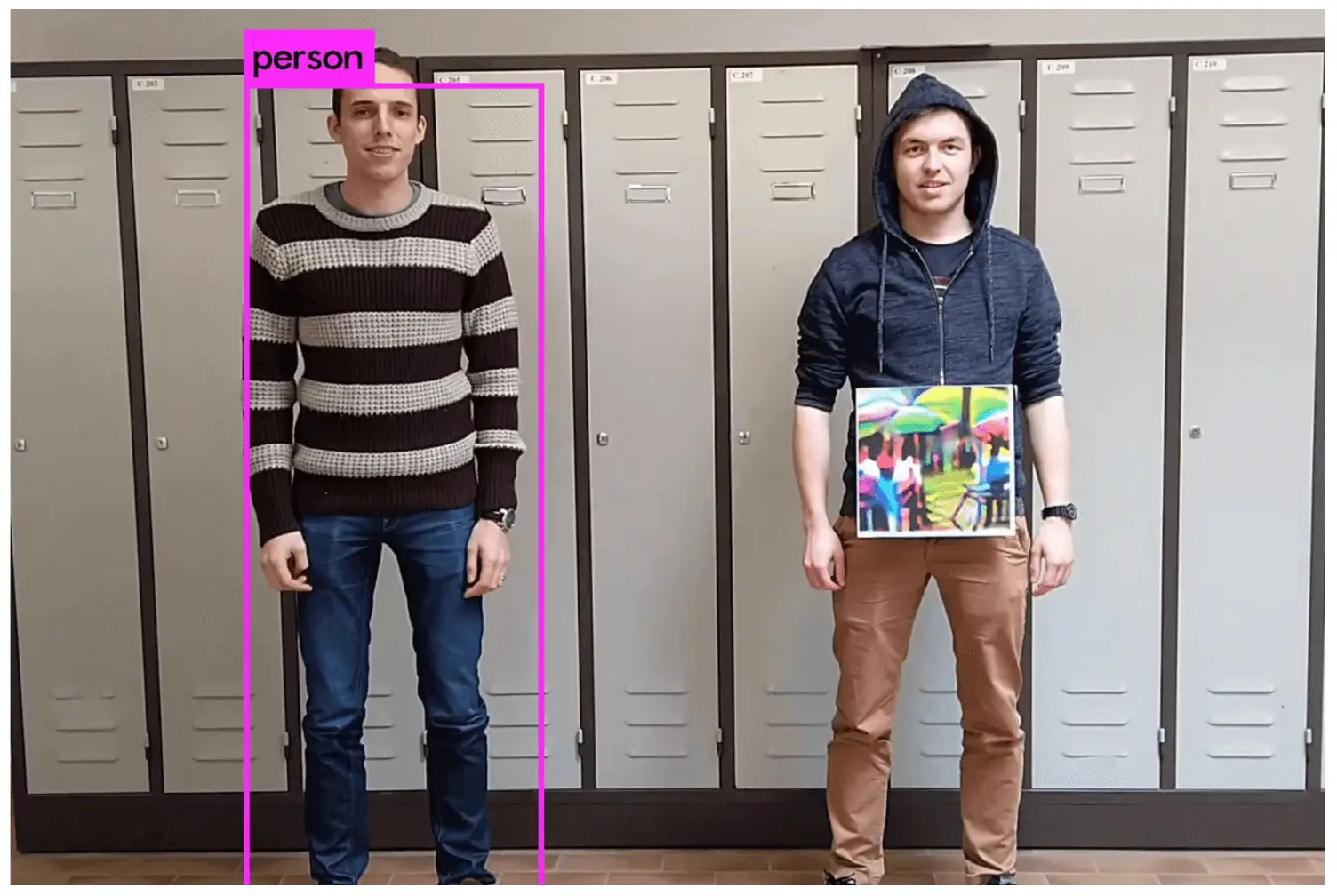
Credits: Is Fooling An AI Algorithm Really That Easy?
Main Threats of AI
AI-Powered Hacking
The history of hacking shows that, as security protocols and tools get more sophisticated, hackers get more creative. Security specialists have started using artificial intelligence a while ago, and hackers are not far behind.
Fighting fire with fire might just be the winning tool for malicious hackers looking to exploit AI security algorithms. Machine learning techniques used for hacking can find bugs much faster than humans or conventional malware. If the security system is powered by AI, the process might take longer. However, the malicious algorithm goes through supervised learning from the security algorithm and fools it in the end. Generally, this approach can be useful without bad intentions in Generative Adversarial Network (GAN).
In order for organizations to secure their AI applications and machine learning models, they must leverage security solutions that provide hyper-secure confidential computing environments. When confidential computing is combined with the right cybersecurity solutions, such as a resilient Hardware Security Module (HSM), it can provide robust end-to-end data protection in the cloud for AI applications – big and small.
Fake News and Propaganda
Major social networks already use machine learning techniques to detect the spread of fake news and hate speech. They might not be perfect, but the future should only bring improvements. The other side of the spectrum has people taking it one step further.
Anyone with a computer can manipulate tweets and images to harm another person’s reputation. However, many fact-checkers can detect these manipulations and shed light on the actual truth. Faking videos and the human voice is a new cybersecurity threat. There are videos of celebrities and politicians talking about things they never actually said. It’s called a deepfake. Check out this video of Obama and just think about the endless, mostly malicious possibilities with deepfakes. Generating text-to-speech with any voice is interesting if you don’t use it to create fake interviews like this one.
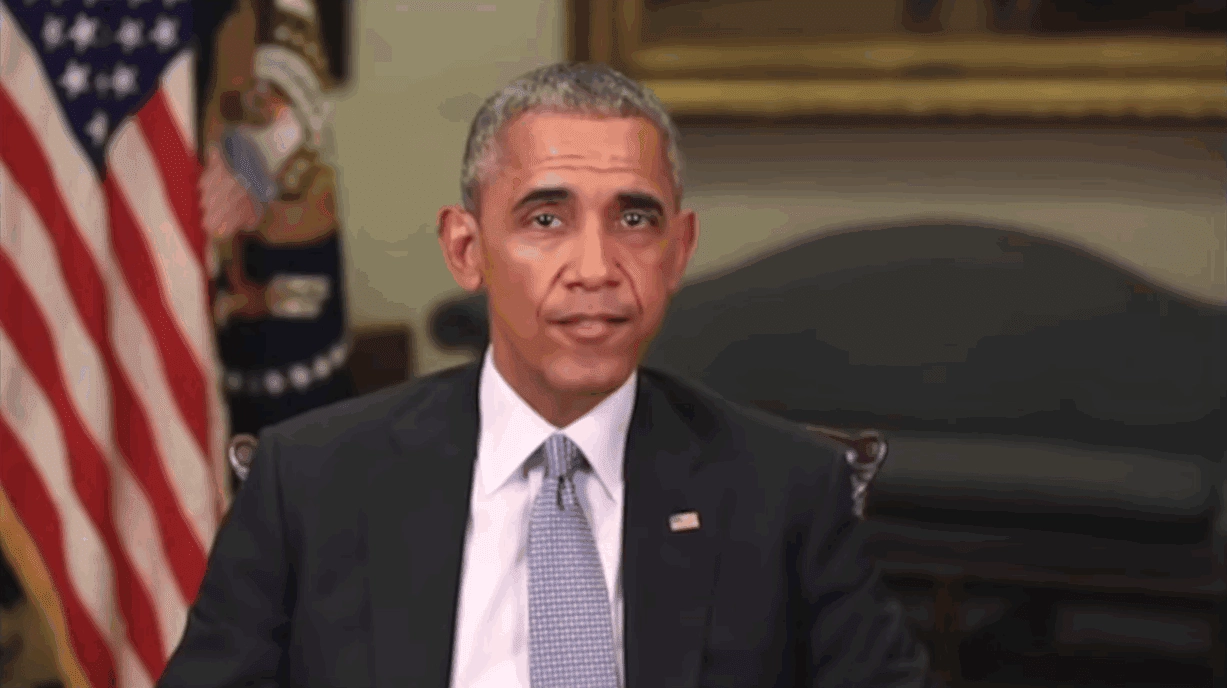
Credits: YouTube Screenshot - BuzzFeedVideo
How Hackers Attack Machine Learning Algorithms?
Not only do we struggle to determine if politicians are telling us the truth, but marketers try to hook us up with all kinds of products that are just what we need because they are better than the competition, the safest, the only one that will get you your desired results. The hyperbole can be exhausting.
We have never experienced such a time when we have so much information and so many opinions thrown at us from so many angles. In response to our struggles, fact-checking organizations that are dedicated to dissect and analyze statements made by politicians and public figures now exist and are becoming increasingly visible.
As data continues to explode, the ability to rummage through it to find the truth required in a situation is essential. Consumers won’t be patient either. They want to find out anything they seek to know and they want to know it now. Brands will have to respond with truth and transparency if they hope to remain competitive.
Businesses are beginning to respond to their customers’ demands for facts. The big data-driven, machine-learning tech that is rolling out gives customers the raw material needed to measure and quantify absolute, objective facts and then act based on those findings, rather than rely on opinions and gut instincts so common today.
Hackers carry out AI attacks in two areas: inference and training. Attacks during the inference occur when the hacker knows a few things about the model. They then try side-channel and remote attacks using the model’s responses to various inputs. Training attacks struggle to change the model by corrupting the input data or the algorithm logic. Here, data mining takes the algorithm in the wrong direction.
Propaganda, misinformation and fake news have the potential to polarize public opinion, to promote violent extremism and hate speech and, ultimately, to undermine democracies and reduce trust in the democratic processes.
There are three attack types hackers use to corrupt the machine learning algorithms:
Evasion attacks - Hackers provide faulty algorithm inputs, leading to incorrect decisions.
Poisoning attacks - Hackers provide poisoned data for training sets. which corrupt the machine learning algorithm and spoil the data mining process.
Privacy attacks - Hackers use the training phase to try and retrieve private data from the algorithm.
Data scientists need to train machine learning algorithms against various threats. It's important to stay safe against this variety of possible hacking attacks.
How to Make AI More Resilient?
Data scientists around the world are working on cybersecurity solutions to make AI more resilient to hacking attacks. Human analysts continually work on new threat detection methods with the help of threat intelligence. Many approaches work towards the same goal. The goal is to prevent machine learning algorithms from being tricked easily. Proposed techniques vary, and they use dimensionality reduction, intrusion detection, etc.
The first advocated approach is to make AI more resilient by training it with adversarial big data. Let's start with an AI (machine learning/neural network/deep learning) model. It trains for potential security exploits by taking data inputs the hackers would normally use. The experience can come in handy to help the algorithm adapt before the actual threat appears. This way, when hackers try to exploit the algorithm, it will be ready and recognize that someone is trying to exploit its weaknesses. There are two ways data scientists and cybersecurity engineers work with adversarial big data:
Thinking like a human: In the Tesla example, AI makes wrong decisions in situations where a human brain would probably guess right. Data scientists incorporate human thinking into machine learning algorithms and deep learning models. The purpose is to prevent them from being exploited using poisoned data.
“The model learns to look at that image in the same way that our eyes would look at that image.” - Arash Rahnama, a senior lead data scientist at Booz Allen
Threat detection before training: The first method still sees AI working with poisoned data that try to trick it. In this scenario, the effort is made to detect potentially dangerous data before it enters the training set. This way, AI uses anomaly detection to recognize various types of attacks rather than types of potentially dangerous data.
“Instead of classifying images, we’re classifying attacks, we’re learning from attacks.” - Rahnama
An ML system is an intelligent, non-human decision maker in an organization, but it is fundamentally different from a human decision maker. Accurately measuring the resilience of such a system requires us to extend our tools to accommodate these unique differences.
Further research is required to develop an assessment of ML system resilience, and it's possible that this research will point toward changing the CERT-RMM itself.
Even if that happens, the overarching goal of the CERT-RMM and its derivative tools remains the same ever: to allow an organization to have a measurable and repeatable level of confidence in the resilience of a system by identifying, defining, and understanding the policies, procedures, and practices that affect its resilience. The fundamental principles and objectives remain unchanged when we consider an ML system instead of a traditional system. If we are to be confident that ML systems will securely operate the way they were intended and for the critical missions they support, it is imperative that we possess the right tools to assess their resilience.
Final Words
Using artificial intelligence in important sectors requires special attention to cybersecurity. In certain areas, AI mistakes are tolerable and even useful for future development. In other areas, mistakes cost money and financial, psychological, and physical damage.
AI technologies are unique in that they acquire knowledge and intelligence to adapt accordingly. Hackers are aware of these capabilities and leverage them to model adaptable attacks and create intelligent malware programs. Therefore, during attacks, the programs can collect knowledge of what prevented the attacks from being successful and retain what proved to be useful. AI-based attacks may not succeed in a first attempt, but adaptability abilities can enable hackers to succeed in subsequent attacks. Security communities thus need to gain in-depth knowledge of the techniques used to develop AI-powered attacks to create effective mitigations and controls.
According to a report on the Future of Jobs by the World Economic Forum, AI will create 58 million new artificial intelligence jobs by 2022. There is an excellent chance that by 2030 AI will outperform humans in most of the mental tasks but that does not mean it will take away jobs.
Data science companies have already tricked many machine learning algorithms, and it’s not like they had such a difficult time. From traffic sign recognition to reading ECG, the consequences of AI mistakes can be rather costly. New cybersecurity threats emerge every day. Data scientists must come up with threat detection systems before they take control.
Fighting hackers is demanding, and it will never end. Some people use deep learning models as security solutions. Other people with malicious intentions use it to go around our defenses. It’s a never-ending war that comes down to who has better machine learning techniques. Big data science companies should invest in fulfilling their cybersecurity tasks. Investments in data science and cybersecurity intelligence are more important than ever. Deep learning models and neural networks can sometimes perform eerily human-brain-like. But they can also fail easily, so we should not fear its world domination in the next couple of years!