How Data Science Solutions Are Fueling Growth of Fintech
Data science in Fintech has reached new heights. Deep learning and artificial intelligence are the techniques that data science relies upon. It processes data and makes predictions about future behavior and patterns. In recent years, data scientists developed several new methods to handle big data.
Fintech improves the automation process and delivery of financial services. The industry is on the rise; room for improvement is there.
History of Fintech
1866 is the origin point of Fintech. Transatlantic cable provided new infrastructure during the period of intense financial globalization. In the 50s, companies introduced new ways of customer interactions through credit cards. The process of digitization started in the middle of the 20th century. It brought us the first digital stock exchange and SWIFT communication protocol. Approaching the new millennium, the Internet made online banking possible.
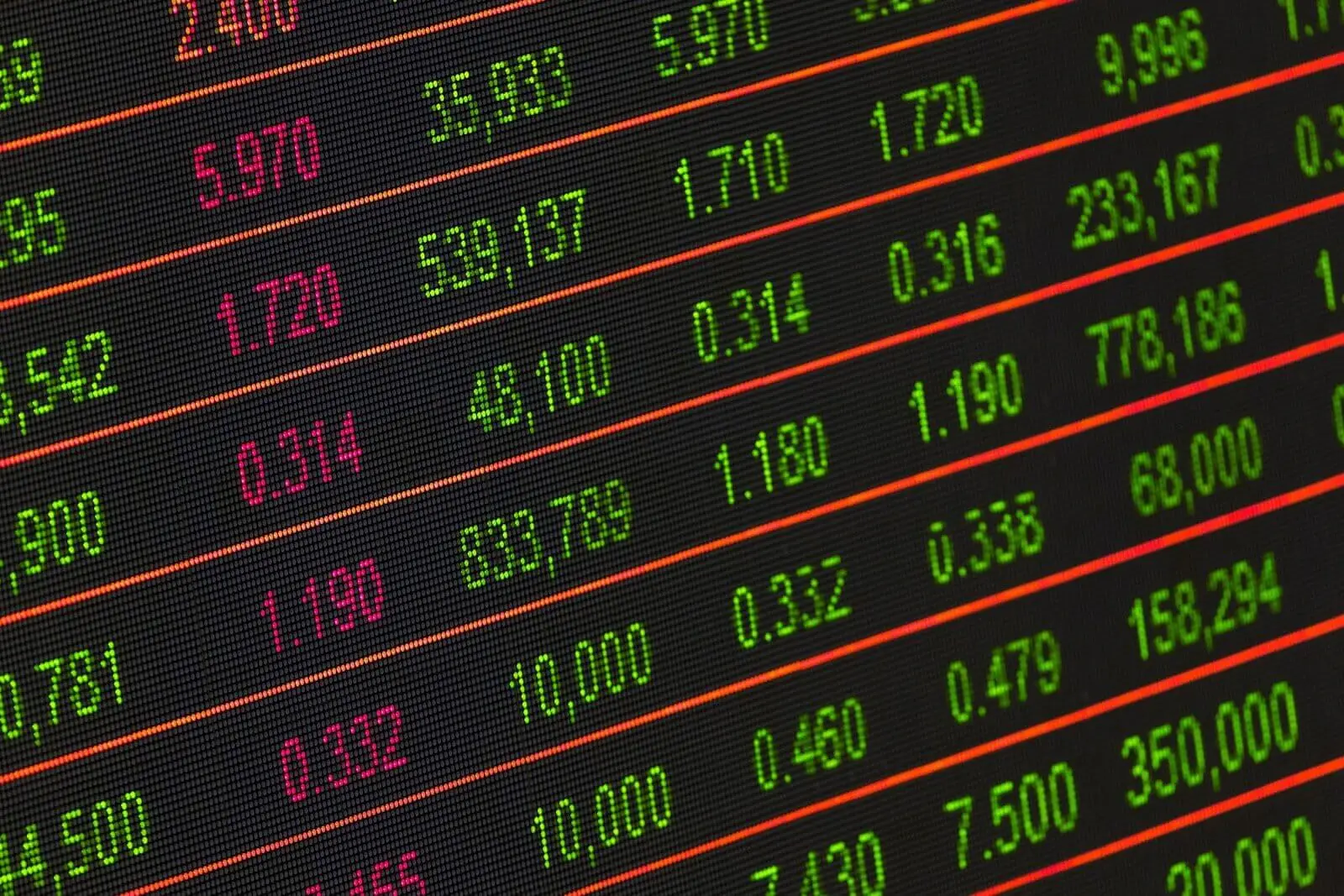
Present Day
The turning point was the 2008 World Financial Crisis. People looked for new ways to replace traditional banking services. In 2009, Bitcoin made its debut, followed by other cryptocurrencies. Apple pay and Google wallet revolutionized the way financial services get delivered.
Currently, there are many Fintech companies in the world, but these are the consensus top 5:
- Ant Financial
- Adyen
- Stripe
- Paytm
- Coinbase
Data Science in Fintech
Fintech proved to be an exciting market for data science companies. The power of algorithms revolutionized the business processes of investment banks. AI in Fintech makes real-time predictions of investment opportunities and spots trading patterns. The financial sector has seen these five changes as a result of data science consulting:
- Transaction and Payments. Data analytics are used to enhance the customer experience and the product's value. Integration of personal data and payment records, loyalty rewards, etc. improves customer engagement.
- Revenue Collection. Predictive models predict the probability of payment at the moment of purchase. Debt collection poses a delicate issue, avoided through optimal strategy. Advanced insights into behavioral economics allow a more successful debt collection.
- Corporate compliance. Detecting early signs of non-compliant behavior is crucial for big companies. Data science empowers engines and architectures that ensure corporate compliance. They result in successful predictions for activities along the value chain. Successful predictions drive better service quality.
- Customer Journey Metrics. Most financial organizations focus on optimizing conversion rates and minimizing churn rates. Deep learning provides a structured analysis of various interaction data like social network activity, unstructured text, feedback rankings, etc. They are improving upon these results in bolstered sales and engagement.
- Credit Evaluation. Most banks want to make credit accessible to more people. That’s why Fintech companies are looking into creating faster ways to evaluate credit risks. The proposed solution is a combination of various data sources and calibration against training data. A good predictive model drives sales to new heights.
Effect of COVID-19 on Data Science in Fintech
The pandemic has put new advancements in the fields of machine learning and deep learning to the test. More companies are transitioning to a remote working model every day. Security and Online Loans proved to be alarming issues that Fintech companies had to provide solutions for due to COVID-19.
- Security. COVID-19 has amplified already existing security and cyber threats. Luring targets to fake websites, coupled with speared phishing attacks, were the choice of fraudsters. According to recent reports, Google blocked more than 18m of such e-mails.
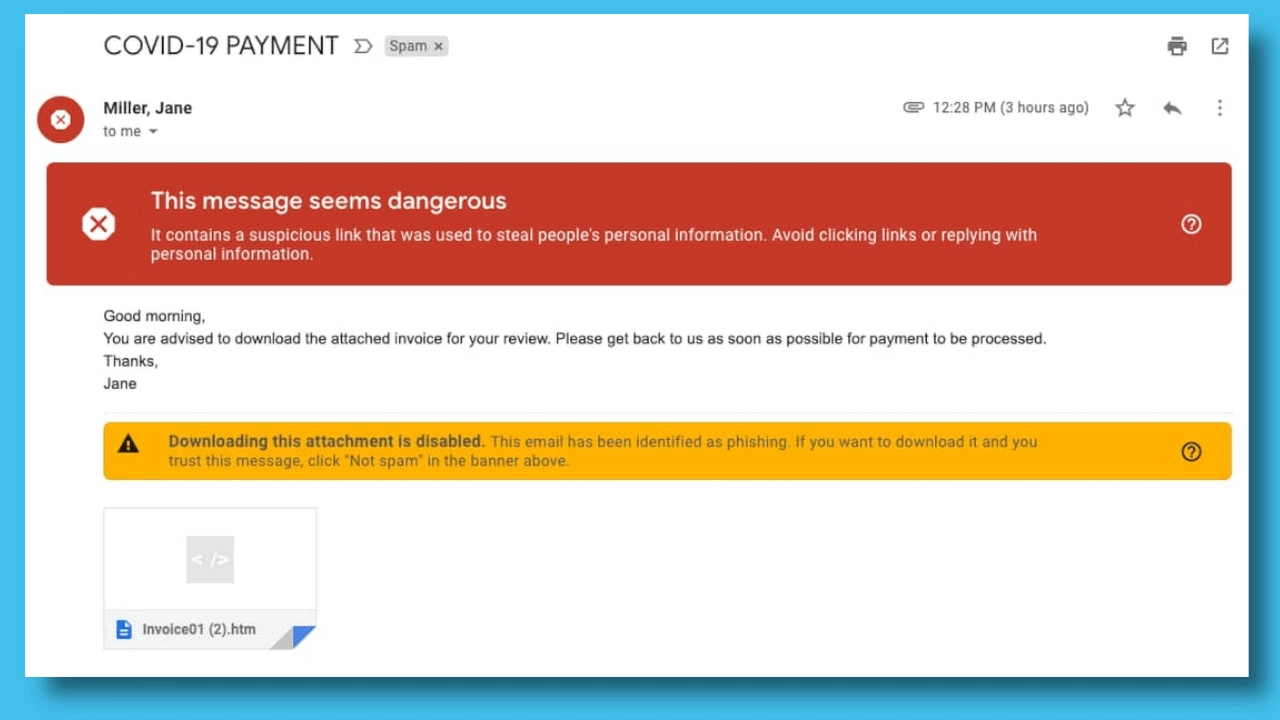
Source: Google blocking 18m coronavirus scam emails every day | BBC
Insider malpractice presents itself as another issue. Home environments are less secure, providing a chance for disgruntled employees to leak information to outside sources. Controls to verify damage, face-to-face contact with agents, etc. have all taken a hit. So the question is, how can AI help Fintech in terms of security? The answer is simple. AI allows organizations to identify suspicious patterns invisible to experts. It performs the task in the following steps. First, AI connects a large number of data points from remote databases. They include social media posts and IP addresses of public Wi-Fis. Using appropriate machine learning techniques, AI can identify patterns.
- Online Loans. Due to economic lockdown, US banks will experience a drop in the growth of all loan balances. Subprime and small businesses have become the primary focus of lenders. The consequence of this is rising default rates and inadequate reserves. Using alternative data provided by data science outsourcing companies are beginning to look more appealing to Alt lenders. Look out for Lending Club and OnDeck, as they will come under heavy fire.
Should Fintech Companies Outsource Data Science?
Following the latest development in AI, Big Data, and Machine Learning, Fintech companies are looking to extend their reach. Most start-up owners have to address an important decision: “Should they develop products in-house or look to outsource?”
First, let us look at it from a financial standpoint. Based on a McKinsey Global Institute study, outsourcing IT services reduces cost by 60% in the US. Tempting as it may be to develop everything in-house, data science outsourcing is the way to go. It allows business processes to run smoothly and does not stress Fintech companies to build business applications.
Big data is essential for the operational efficiency of Fintech companies. Understanding what products and services customers prefer is achievable utilizing deep learning. Spending patterns depend on the following:
- Age
- Gender
- Location
- Social class
- Purchase history, etc.
All of this sums up to a massive amount of data difficult to analyze in-house. It is the reason why data management outsourcing is a better option. Delegating tasks strategically is one of the ways Fintech start-ups can save money. Companies are often understaffed and looking to outsource data science projects to third-party providers. This is an excellent strategy to call upon before finding an investor. Data science outsourcing may seem like an extra cost, but it saves money in the long run.
Outsourcing data science projects ensures you work with verified experts in the field. Finding the right talent at an affordable price is extremely difficult. Building an in-house team requires a lot of time and money spent on employee reorientation. By working with data science companies, you essentially get an off-the-shelf product ready for use.
Summary
While it has been here for more than 150 years, Fintech has only recently gained recognition as a standalone branch of technology. It is a field full of promise and room for improvement. Recent developments in data science make it hard to ignore all the benefits Fintech can enjoy from them. Fintech is responsible for making wire-to-wire transfers, online banking, and overall faster business conducting possible. On the other hand, data science helps Fintech services run at a smoother and improved rate.
In testing times as these are, the security of Fintech services has been put to the test. Finding that intricate balance of flexibility and maximum levels of security is a challenging endeavor. Recognizing these patterns is made easy with powerful data science tools.
Handling significant amounts of data is the primary issue that all financial businesses have. Doing it in-house is slow and requires a vast workforce. That’s why many Fintech companies look to outsource data science projects. They are simply too complicated and time-consuming to handle.